Making ethics actionable in digital health
Shubs Upadhyay (00:00)
On today's episode of the global perspectives on digital health podcast, I'm very, very excited to have a Titan on digital ethics. Jess Morley of Yale university, and I'll let her introduce herself and her work. but this is a really, really hot topic. We're to be talking about actionable ethics in digital health. particularly because there's so many things that are going on right now
The UK government has just released their AI action plan and Jess has got some pretty spicy takes on this There's the US context right now with what's going on with DEI policies, for example, with Meta, allows us to kind of reflect on the ethics space in general.
And also just with the proliferation of LLMs and AI and thinking about the LMIC context particularly, I think there's a lot to extract from Jess's knowledge and experience in this topic. So I'm really, really looking forward to take away some actionable things that we can take back into our own work
What does this mean for underserved populations, both in low and middle income settings and high income settings? It's going to be a juicy episode, I'm sure. So let's get into it.
Shubs Upadhyay (01:08)
Jess, it's such a pleasure to have you on the Global Perspectives on Digital Health Podcast. What a time to be a professor of digital ethics. we've got some juicy topics that are literally just steaming out of the oven right now, including the AI action plan from the UK government, crazy stuff going on in the US with DEI and...
company, big tech U-turns on their policies, etc. given the political environment. I think there's lots and lots of insights that you can give to policy folk, implementers and vendors into how we can make ethics actionable. Let's start with maybe an intro about your background and yourself
Jess (01:46)
Yeah, well, thank you so much for having me. I'm really excited to have this conversation. Also, thanks for promoting me. I am postdoc, not yet prof. That's the aim. We're probably not there yet. So currently, I am postdoctoral research associate at the Digital Ethics Centre in Yale University. Every university has different terminology, but essentially, I am immediately post PhD.
Shubs Upadhyay (01:56)
Okay. okay. I put it.
Jess (02:11)
But I have been bouncing around in this space for a long time. Very originally, I actually worked for the UK government. I worked for the NHS for about five years in a variety of different guises. When I first left university and I thought that leaving was going to stick, that was a big fat lie, but at least thought at the time this was a thing. So I went and I worked for the UK government in a variety of different roles, as I said.
mostly within the NHS tech space. and then the most late latterly role I had was in NHSX as what was the sort of AI subject matter expert for health and care. That was in 2018, 2019. And then whilst that was happening, I just started really thinking a bit more deeply about the implications of the types of things we were trying to make policy about.
And I slowly, I suppose, started to feel that there was a lack of depth and nuance and understanding in the policy domain, including within myself. And we were therefore a little bit vulnerable to manipulation by big tech. I couldn't really find anyone at the time who could tell me, you know, this is how we both make the most of these amazing opportunities. But this is also all of the sort of really
risky things that you should also be thinking about from a sort of ethics. And I suppose at that time, my head would have been more thinking about sort of social implications. And my response to that, therefore, was I'll go back to school. So I went back to university, I went back to the University of Oxford originally to do my masters. The plan was that I would do my masters over two years. So I'd do it part time while still working for the civil service. Halfway through that period of time, we hit 2020. We all remember what happened in 2020.
Shubs Upadhyay (03:37)
Mm-hmm.
Jess (03:59)
So I left the civil service, I started working for the University of Oxford in what is now the Bennett Institute for Applied Data Science. We did a bunch of stuff, including building this big platform called Open Safely and my boss and I at the time, so Professor Ben Goldacre and I wrote the Goldacre Review. Then in the middle of all of that as well, towards the end of 2020, I started my PhD. My PhD is...
Designing an algorithmically enhanced NHS that is the short version. The very long version sounds extremely Oxfordy and makes me want to punch myself in the face. So I just give the short version. But essentially I looked at how do you deploy what I call algorithmic clinical decision support software into the NHS from four pillars, which I think will cover and topic of this conversation. So technical feasibility, legal compliance, social acceptability and ethical justifiability. I finished that.
Shubs Upadhyay (04:31)
Hehehehe
Jess (04:49)
in January last year, so one year out and I immediately moved over to Yale. And as I said, now I'm a postdoc at the Digital Ethics Center, so that's me.
Shubs Upadhyay (05:00)
Superb. What a rich set of experiences and expertise that you're building. So before we get into the questioning, I kind of just wanted to set up some, maybe some context, at least from my perspective, having worked both as a clinician and also working within digital health, with a digital health company. So from the vendor developer perspective. To a lot of people, ethics is kind of a,
nebulous fuzzy concept and essentially, especially from in certain ways of thinking about it, it can feel like a tick box exercise. So hey, we've got this course, the WHO have a course, you do it and you're like, tick, like ethics is done. And actually, people have really good intentions around it. And yes, this is our mindset. This is how we're going to do it. We're going to create these ethical principles.
Let's go for it. This is great. It's really important. And also that's part of it. And then you can communicate to the world, hey, aren't we so great with doing ethics like amazingly, but in a way like the most ethical ever. And it's just like my view from the industry. And then what happens, I think, which is the key thing is these intentions bump up against like business realities. So product prioritization conversations.
hey, this is not a business priority right now, we really want to carry on this, you know, this feature or this initiative that will really improve some of this, we're have to kind of like kick it down the road a bit because, you know, we need to do x, y, z, which are like way more important right now. And this can happen in terms of, this thing that we should do that's really ethical, but and other priorities have come along, or from the other direction.
hey, we're going to do something that's a bit ethically dodgy, but we're going to justify it with all this like wrappers around. And so for me, ethics feels really, it's hard to kind of break through that because ultimately, you know, vendors are like business entities. And so I think what I'd really, really like to understand, at least from that perspective is
Jess (06:39)
Mm-hmm.
Shubs Upadhyay (06:57)
how can people with good intentions negotiate these things in a really good way? How do you make good decision making that elevates this? And how does the industry elevate this so that also on the other side, there's like pull factors from the industry? And so for me, that's really, really what I want to cover. And hopefully we can move from this like performative optics side of ethics to like actually we're moving forward because I feel like we're not
moving forward, at least from what I see in terms of having actual tangible outcomes on equity and all the other principles and pillars of ethics that we see. I think first, before we start, some definitions to really get aligned on. So why don't we start in this space and discuss your definitions around some of the important concepts when it comes to ethics and digital health.
if you've got a really good way of summarizing that, that'd be really useful for people,
Jess (07:49)
Yeah, so I think for me, ethics is primarily a way of thinking. And the best way I think you can sort of clearly understand it, actually is a metaphor that my prof. Luciano Floridi uses all the time. I think it's probably the best one. If you think about it, law being the rules of the game and ethics being how you win. So ethics is really that sprinkling on the top.
of how you make sure that something doesn't really massively backfire in a way that would be damaging to society, but also damaging to your business model and all of that type of thing, which is all of the type of stuff that we'll talk about more in this conversation as we go on. So I won't rattle on about it right now. Within ethics, then there tend to be...
The main way in which people get into it, at least in this domain, in the space of sort of digital health or technology anyway, is by talking through different principles, the most common of which are beneficence, non-maleficence, autonomy, justice, and explainability. So very, very briefly, beneficence is essentially do good. Non-maleficence is effectively do no harm. Autonomy is...
protect the individual's right to determine their own life. Justice is a complicated one and people get really controversial about what that means. They sometimes they think it means diversity, sometimes they think it means fairness. There are upwards of 33 different definitions of fairness. And so it's very complicated, but normally in the space of health and I know bearing in mind where we want to go in this chat, there's sort of two pillars of
of justice tend to be sort of equality and equity. Equality basically meaning everybody is the same. We make sure everybody gets the same sort of outcomes or really we're talking about same access and equity meaning everybody gets what they need in order to achieve the same outcome. And those things are sort of two different sides of it, but that's really what you want in that sort of pillar of justice. And then explainability, the sort of fifth one.
is really about, you understand what an algorithm is doing? And again, there are different ways within interpreting that, but that is essentially what it means. Then there are some sort of subsidiary terms that often get banded around accountability and transparency are probably two of the main ones. Accountability is essentially who do I put the point of the finger at if something goes wrong?
And transparency is really the mechanism that enables that accountability. So how do I know who was the decision maker at different points in time in order to enable accountability? And then the sort of last two that I think often come up in these types of conversations is really validation and calibration and sometimes evaluation. So validation would be essentially
Does the thing do what it says it does? Have we tested an algorithm outside of a lab? And bearing in mind, a lab is essentially a computer in a different environment and sees that it actually works. Calibration is normally what we're talking about, local calibration. So does something then, what are the tweaks that need to be made in order to make something work in a specific local context? And evaluation would then be.
what has happened as a result of that algorithm being used in real time. So those are very quick fire definitions of the most common concepts that I think come up in the conversations about AI ethics and digital health.
Shubs Upadhyay (11:27)
Thanks, Jess. That's really useful and I think important to kind of just level set everyone. Lots of people that might be very familiar to lots of people and it might be new to some people. So thank you for going over that very, very clearly and succinctly as well. Okay, armed with that, I'd love to get your take on where the industry's at right now.
And of course, as we mentioned, we're literally, you know, a day after the UK's action plan on AI. There's lots and lots of stuff coming out of the US what's the US government, you know, what the new government that's coming in, like, what's their take going to be? Where's the FDA fit in all of this? What, you know, what's going on with quality assurance labs? And also just zooming out.
no pun intended meta level. You've got, you know, not within digital health, but you've got organizations like meta, who have taken complete U turns on on their DEI policy, for example, based on like political determinants of business decision making. So to me, all of that kind of feeds into my questioning around what's your take on the state of the industry? Where where are we at? And what you know, if you
you've recently on LinkedIn, very, very pointed article kind of giving your first interpretations of the AI action plan as well. So feel free to kind of talk about some of them because some of them are really great.
Jess (12:44)
Yeah. So actually, I think the two things go, go hand in hand actually, because the AI action plan, what it reflects, at least from a rhetorical perspective, I think is a product of where we are from an industry perspective with regards to ethics. And my main view in that is I think we've gone backwards. So I think if you went five years ago, and then three years ago, even the conversation around
ethics, DEI, equality, equity, all of the things I've just defined was much higher. It was a much more prominent in policy and by policy, mean, big P policy and small P policy. So big P policy, the stuff that was coming out of governments and little P policy stuff that's coming out of individual companies. It was very much being positioned as a strategic advantage to be being seen as quote unquote,
pro-ethical. And we can talk about whether or not that was really working in a minute, but just from a sort of conversation perspective of narrative flow, that's really where we were. It was seen as being a strategic advantage. You had conversations coming out from the UK government saying, we're going to win the race by being the most ethical, by being the best place to come and do ethics. had, like you said, you've got the big companies making huge statements with regards to
their sort of ethical principles. And you're absolutely right. That was everyone from Google and Meta right down to like your big pharma companies like Roche. They have an AI ethics principles. You had that big document that came out from the WHO. So it was really foregrounded in those kinds of conversations. And that was also the context in which we did, like I mentioned before, the Goldacre review that was 2022.
The big thing in that whole sort of concept, the core concept essentially we were being asked then was really how do you enable public good to result from the use of health data while maintaining privacy? That is essentially an ethical question. Because I didn't mention privacy in my spiel, but that's mostly because everyone is already familiar with it. Now, if you take this to where we are now, the vast majority of that has gone away.
Shubs Upadhyay (14:46)
Mm-hmm.
Jess (14:58)
we have really pivoted more towards this idea of what we want to achieve from AI is economic growth. That is our sort of big strategic aim. And now you see that very clearly in the opportunities AI plan. For one thing, it's literally called the opportunities plan. There is nothing in there about balancing
And so that was really what I was saying on LinkedIn is that the rhetoric and the of the thing that it's saying is essentially, we don't really care so much about how you balance the opportunities with the risks. We just want to go hell for leather on making sure that we capitalize on the opportunities. Whereas what I would normally want us to talk about is the dual advantage, which is how do you capitalize on the opportunities whilst proactively mitigating the risks? That sort of second part of the clause has gone.
You also start to see this real thing now of where, as I was saying previously, the competitive advantage was, can we do things in the right way? The competitive advantage now really seems to be how much do we build in-house? So again, you start to really see that in that AI action plan. It's all about sovereignty. So it's all about how do we, the literal words are, how do we be an AI maker instead of an AI taker? Which is how do I,
make sure that everything is built from inside my house. The thing is also we are on the side of the innovators. And the point I made in LinkedIn was why are we not on the side of society? And this is not a UK only thing. You see this reflected in US policy. You're also seeing it reflected in, like you say, small P policy in companies. Like we have now seen these whole conversations coming out with social media platforms.
Shubs Upadhyay (16:23)
I saw that,
Jess (16:41)
reneging on their promises with regards to misinformation or disinformation under the guise of protecting free speech. But then you've also got, like you said, you've got meta coming out saying that they're sort of deprioritizing their DEI strategies. it's so that whole thing has flipped and it's really happened in the last 18 months, I would say you've started to have that effect. And I think there were two reasons.
I don't like to talk about causality because that implies that it's linear. But there are Two sort of drivers, I guess, of where I think this is happening. The first one is that I think it's post-COVID, post-COVID economic slump, and people are now just desperate to make money.
Shubs Upadhyay (17:10)
I was going to ask, so thanks for preempting.
Jess (17:27)
and get out of that and to start producing again. I think there was this whole feeling where for about three years, effectively the whole world was on hold. And now it's like, we want to grow, we want to grow, we want to grow. Then I think you've also had the other one is this sort of big shift in terms of political shifts. You you've had elections across the world that have very clearly shifted towards the right.
That isn't what happened in the UK, but the UK is trying to be competitive in a post-Brexit world. And that means it needs to keep up. And so that, think, is the second one. And then the third reason really is generative AI. I think generative AI came, and whilst it definitely didn't surprise the techies, I think it surprised the world. And that excitement and enthusiasm, because it is perceived as being magic,
I think took off the brakes in people's minds of what AI can do. I think before that people were a bit like, yeah, I can see some of its uses, but I don't see it necessarily changing the world. And therefore I'm okay with there being some friction in the system. Now it is because of generative AI, because people don't understand what it is, because it seems magic.
They're like, we want to go and grab this by the horns in whatever way we possibly can. So that's really the sort of reasons contextually, I think we've seen this big flip.
Shubs Upadhyay (18:52)
Yeah, I can see that. so main takeaway, we've gone backwards. And we've moved from kind of being, you know, pro ethical and performative to, actually now, we need like, it's economic growth at almost all costs. And that's the key priority. I definitely see that in many other types of conversation as well, not just with, with ethics, but, you know,
Jess (18:55)
Mm-hmm.
Shubs Upadhyay (19:14)
people very focused on, or the industry very focused on short term ROI. And therefore not thinking that joined up around the bigger health outcomes that we're trying to achieve and how we get there. And so, so I reflect that, yeah, definitely the macroeconomic climate is a big driver of that. And this seems like a manifestation of that totally. And also just generally FOMO and vibes as well.
Jess (19:18)
Mm-hmm.
Shubs Upadhyay (19:38)
So, okay, that was such a great summary. if you haven't read Jess's LinkedIn article, I'll put it in the notes. And there's some really, great hot takes in there. You mentioned one, which was around supporting innovators and actually like flipping that to supporting society. And for me as well, know,
Ethics helps you make good decisions and that's what you want it to do. Right. And so, you know, this is going to be driving government level and other organizations decision making. You create the system and the selection pressures in the system. And suddenly, yeah. At the end of that, yeah, well, economic growth was your goal. what is that going to look like?
in that system in that evolutionary system? What other mutations are we going to see? It's going to I think it's going to be really interesting. One thing that stood out to me, Jess, there were talked about data sets. I was surprised to see that there wasn't anything about specifically going out to find and make sure that data sets were inclusive or curated specific parts of the population. I was surprised not to see that.
Anything else that you want to talk about on
Jess (20:32)
No, so I completely agree. In fact, one of the things I was commenting on was the fact that there are several references in the, they're not even references, they're direct recommendations to, we want to go out and get more data. We want to prioritise data. And actually, this is a really good conversation to sort of re-empt the point we just made. All of that in there, what it says is what we want to do is prioritise the collection of data that is the most valuable.
First of all, I hate that whole narrative. think this idea that you assign value to data before you even decided what you're going to do with it is literally nonsensical. But let's put that aside for a second. It's all about let's collect the most valuable data sets. Whereas really, if you were going to do data curation, and I have been banging on and wanting the government to pay more attention to data curation for years, is, OK, so where are the gaps? Not from a
value perspective, but where are the gaps from the people who we do not have represented in the data sets? And there are people who are completely invisible from a government administrative data perspective. And then there are people whose parts of their lives are not reflected in the data set. So ideally, think if you were going to go in this direction, I would want to see sort of strategic attempts to go in it and fill in those gaps.
But it's also very similar in the sense that there is a sort of almost like a sort of wink of, we have to put it in somewhere, reference to we must increase the diversity of the AI workforce. all it says in there was the fact that 22 % of the AI workforce is women. OK, yeah, that's not great. But that is also
Shubs Upadhyay (22:06)
So you call that one out, yeah.
Jess (22:17)
a product of the wider pipeline, less women go into data science in general. That's largely because I think the way that maths is taught in schools is deliberately unappealing to women. But there's a whole thing. But that is a tiny, tiny, tiny fraction. And I'm saying this as a woman, but it's like it's a tiny fraction of the meaning of the word diversity. It's got nothing in there about the fact that, you know, you also want people with different
varieties of lived experiences. That means you need to have diversity from the perspective of sexual orientation, but also to like gender expression and people of color and people who have different neurodiversity experiences because there are all of these different things and then the points of intersectionality. So if you want to design a diverse workforce, you don't design it so that you have 50 % women and 50 % men. That is not a diverse workforce.
You want to design a diverse AI workforce from the perspective of the fact that we are designing tools that affect every single person's, every single aspect of every single person's life. Therefore, the sort of fundamental point of diversity has to be making sure that we have diversity in terms of lived experience. And I think that was really, really, really missing. And again, I think that had this thing been written three years ago, maybe.
there would have been more of those conversations in there. Instead, everything has been whittled down to sort of metricized conversations. And because the other one we haven't mentioned yet, but we should, I suppose, because it's the elephant in the room and people will yell at me if I don't. The whole conversation is now about safety. Well, instead of about ethics.
and people will say, no, they talk about making sure that the AI Safety Institute is beefed up. Yeah, great, but safety is a tick box metricized thing. It has got nothing to do with the wider conversations with regards to sort of social implications, but it is easy to achieve and it's easy to put in your budget.
Shubs Upadhyay (24:24)
I think what we've navigated so far in the conversation is to break it down into two manageable chunks, one is like the policy determinants of how do you elevate good outcomes in terms of equity and the other ethical principles that you mentioned.
Jess (24:27)
Mm-hmm.
Shubs Upadhyay (24:44)
The other kind of stakeholder that's key in the room is like vendors and the builders. And so you've mentioned big tech and some examples of that.
what's your take on how we can get ethical principles?
Just like from being like a nice to have but to like, how do you make this a business priority for a vendor? What's your what's your thought on this in terms of Jess' state of the industry?
Jess (25:06)
Jess' state of the Industry. So I think there are a few different ways of talking about it. The first one is the reason why people, think, especially vendors and especially SMEs, are sometimes reticent. And I know this because a few years ago we went out and surveyed people and we spoke to them and did interviews. And I was basically like, what is it that prevents you from doing these things?
And the number one sort of comment was it's expensive. And then it eats into the bottom line. To a certain extent, I have empathy for that. And I think from the perspective we mentioned briefly before, with everything being about ROI, I do think there is this underlying fear that we are in the middle of an AI bubble that is at some point going to burst.
and all of the investment is going to drop out of the bottom. And I think therefore people are keen to avoid that by turning around big and fairly rapid returns on investments. And that return on investment tends to be interpreted as economic return, not just not sort of a wider, more social definition of value. So I do understand that and I don't.
I'm not coming in and trying to bang people over the heads and be like, you must do this. And like, you can't operate like a normal business. That would be not good. I also should say that I don't believe in role-based ethical probity. I don't have as much as I am a sort of massive champion for the public sector and sort of civil society and have spent the vast majority of the last 12 years championing the NHS. I don't believe that public sector
automatically good, private sector automatically bad. I think there are actual blurs between those two lines. And we shouldn't immediately be wrapping people on the wrist just because they want to make money for their investors. Where I think there are ways in which we can change the conversation is in a couple of words. The first one is
I think we have to get away from this narrative that the idea that ethics is just an extra thing. You can build ethics into your everyday practices without it necessarily being seen as an extra thing. For example, there are lots of good practices that are just in actual good software design and doing good pragmatic software design that you can see as being
ways of supporting ethical principles. If we are talking about things like making sure you have representative data sets and making sure that things perform equally for everyone, there is a way of seeing that as being really annoying and like, gosh, this is extra expensive. I have to go and collect data. There is a different way of seeing it, of that being like, well, this means I get to sell my product to more people.
Yes, maybe that's annoying to have to go through and jump through those extra hoops, but those are also actually principles that just come from best practice in software design. All we are doing is now expanding them to say that we also want that to have a degree of sort of social element on that.
So that's sort of number one. And often what I talk about is how do you get to the MVEP? So how do you get to the minimum viable ethical product? Yeah, then we can level up. Then we can go through and jump through hoops. But actually, what are the things that really just mean we don't treat things as a sort of exceptionalism, AI exceptionalism. We don't just let them get away with it just because it's AI. And so you build those concepts into
your everyday business as usual, rather than seeing it as an extra category of thing that you need to do. The second thing then is, yes, I do think policy has an enormous role to play in this space. And this is the point I made, I think, briefly at the end of my post on LinkedIn about the AI opportunities action plan was, yes, obviously the proof is ultimately in the pudding.
most of the time what's going to matter is what does the government actually do? So what does this translate into in terms of where does money flow? Where do we see the development of standards or guidelines or procurement practices? All of these things are signals or they're what you might call more formally levers that enable a sort of way of
changing people's behavior who are operating in the environment. But it's not just about that. Rhetoric actually has an enormous influence. Sometimes people sort of roll their eyes when I say things like that and say things like that. Jess, you're sounding too much like an annoying social scientist. What are you talking about? But rhetoric has an enormous amount of shaping power. Because if you think about it, that is how people go in and pitch.
Shubs Upadhyay (29:35)
You
Jess (29:42)
That is how people try and win contracts with the government. That is how you get grants as an academic researcher. That is how you sell your product in terms of advertising spiel. All of that is rhetoric. And I think we have lost good shaping rhetoric from the government with regards to saying that these things are what we want to see. And I would like to see some more of that coming back in because even without the sort of
harder levers, that sort of softer shaping is really important. And the third one, if I just want to be, if we want to be super skeptical, at the moment, we've framed this entire conversation really about it being a nice thing. So like go and have ethics and go and have sort of good principles and practices in order to achieve better outcomes. And that's very positive way of framing it.
the more skeptical, cynical way of framing it is this also stops you from having egg on your face. And this comes back to my definite, my sort of point I made at the beginning of that difference between the rules of the game and winning. The clearest example of this I can give is with the government's repeated mistakes, first of all, with care.data and GPDPR. So for those who listening who don't know very, very briefly,
Shubs Upadhyay (30:45)
Winning,
Jess (31:00)
There was a project back in 2013 that the government wanted to pool everybody's electronic health records and make them accessible to innovators. There was massive public backlash. They paused the whole thing. Then in 2021, they decided to try and do the exact same thing and the exact same result occurred. And now we are seeing the exact same conversation coming up in this idea of let's create a national data library. Now, let's think about ethics versus the law. All of those projects entirely legal.
There is nothing in there that is saying that is illegal. They can 100 % do all of that. Where they failed is in what people who are social scientists like myself call the social license. They failed to understand that actually there was a reticence from a social perspective to that idea. And now understanding that social reticence, that is where ethical thinking comes in and ethical foresight analysis can help play a role.
So if you really just want to strip it right back to the sort of cynical context, it is also about making sure that companies and governments understand that if you do these types of thinking, the types of activities that I'm saying, you build them into your everyday practices, you don't see them as being extra costs. You ultimately see them as risk mitigation. It's a risk mitigating strategy to prevent you getting egg on your face.
and chilling effects and then people disinvesting in you because they think that your product is failure and nobody wants to buy it.
Shubs Upadhyay (32:34)
I really like that. And actually, if I relate that to the times within my own experience the times when it really worked was when we used it in, know, pre-mortems, like risk storming, know, sessions where clinicians were talking with designers and engineers about, hey, we're going to be deploying in this context, applying, from an equity as a quality pillar perspective, how does this apply?
What could go wrong here? Therefore, what do we need to do? I see a line towards being able to operationalize and justify, like internally, you want to justify it as an ROI Like, why, do we need to spend effort on this? because this within the machinations of a company that's like, might be leaning into
building good quality products through a QMS and through regulation, etc. It makes sense, right? And I take that away that actually, the reason it's a business priority is we're trying to make sure that we create a great product that the users really like using clinicians really like and trust.
And they're like the health system will actually say, hey, after a couple of years, hey, that was great. we want to keep you guys because you get it. I reflect that, yeah, actually, that's the way to make it a business priority. So thank you. so we've talked about, we've talked a lot about this UK context and we've come into the US context a little bit.
I think there's a lot of things, know, if I'm you know, health minister or chief digital officer in a country, LMIC country, and I'm looking, I'm observing what's going on in the US, I'm observing what's, I've had a good read and probably had a read of Jess's hot takes on the opportunity plan. Hopefully some of them will now, or already have.
Jess (34:07)
I doubt that video.
Shubs Upadhyay (34:13)
And in terms of how do I apply this to my context as a healthcare decision maker or to my healthcare system? What's important here? What principles do I need to take? Can we go into like a 101, like applied into these types of contexts? And I'd love for you to like, you touched briefly on like social acceptability as well.
I interpret that as cultural understanding and cultural context. So if you could weave that into like your thoughts on this, that would be great as well.
Jess (34:45)
Yeah. so first of all, I should sort of declare my positionality. I do come from entirely either being in the UK or in the US perspective. And I wouldn't want to talk on behalf of people who come from different countries with different experiences. But that set aside from my view on this, there are
couple of things. The first one is really just avoiding falling foul of any narrative that AI or digital health is the solution to improving equitable access. Or that it is the solution to getting away from issues to do with bias, by which I mean like discrimination by clinicians.
So sometimes those are two things that come up particularly in LMIC contexts or even more rural areas of more developed countries. This idea that AI will solve the access problem and that it will solve any form of sort of discrimination because AI is going to be more objective than a human. So sort of 101 is avoid that type of thing, which actually then leads me to my
bigger point and my sort of bigger ethics 101 if you are in a different context. And the biggest one I always say is think critically. So actually just boil this down to a basic set of questions. And those questions are why is AI the right solution to this particular problem? And could this be solved with a different tool?
The second one is what resources do I need in order to actually make this work? And then sort of paired with that is also would those resources be better spent elsewhere? Sort of clearest example of that is there is no point having a very fancy algorithm that does really great diagnostics and will then predict the best type of treatment for that person.
if those treatments are actually not available in the context within which you are deploying the system. And in that scenario, you might actually be better off investing in just buying the drugs that the algorithm would be recommending. The third one then of those questions is who decided, right, who decided that AI was the right solution for this? And that is really where I'm starting to get into that cultural context point.
to sort of weave that in a little bit with regards to social acceptability. What I really mean by social acceptability is are people happy to hand over a specific task that is to do with healthcare to an algorithm? And that is actually going to be hugely culturally variable. Let's just put it in for, because people forget
that actually things like definitions of illness and disease and health and sickness and aging is all culturally specific. And then the ways in which we deal with that is also all culturally specific. So, you know, think about things like how we handle death and dying and conversations around that. That varies enormously. There may be some cultures where it's really, they're really happy.
for there to be a digital mediator with regards to conversations about things like end of life care planning. There will be other cultures where it would be even completely inappropriate for those conversations to be had by a doctor, because people assume that the only people who should be having those conversations is either a religious figure or people from your family. And they would be therefore really antagonistic to the idea of them being a digital mediator.
So therefore it's where was the decision made that this was the right solution for that particular problem. And then the sort of next basic question is who does it work and who does it work for? So does it work in my context, both in terms of my population, but also in terms of my wider system, so my wider care plan, what is available as a resource team?
And then the last one is this sort of what is the long term impact of this? And is this actually going to help me achieve sort of my wider strategic goals? And the way I frame this sometimes is to be more formal is that form follows function. So we often lose these conversations and we assume that we just want to adopt everything adopt, adopt, adopt, adopt, adopt is the best.
thing we want to achieve, rather than having a conversation as, what is it I want to achieve with my health care system? What are my main priorities? And then work backwards from there.
Shubs Upadhyay (39:27)
I
And I think that's a big thing that's missing. I think all over the world, like in all of our contexts, actually, what I observe is that AI has become a goal in itself.
Jess (39:37)
Yes.
Shubs Upadhyay (39:39)
the goal. So when you when you talked about number three, who decided, particularly with a lot in what I observe in the LMIC context is actually it was like a big donor who decided AI should be we're going to fund LLMs going into this context. And what wasn't thought of was, hold on, what are we trying to do here?
hold on, let's take a step back here, like health, like what are the health outcomes first. But because of, you know, economic factors, FOMO and vibes, it's suddenly, this is like the biggest opportunity in any generation. It might be
But it's only if we do it right and we think about it in the right way
Jess (40:17)
Exactly. And it's also what are the sort of knock on effects of that type of reasoning, right? So if you take exactly, like you say, huge amounts of investment in LMICs with regards to AI being interpreted as predictive models trained on electronic health records, well, then you have to go into those places. And you say the first thing they realize is that there is not an electronic heath record.
So then you have to get in the company who is going to build the electronic health record. most of the time that company is somebody who's come from the UK or the US or the EU. They will build that record in a way that reflects their health care context, not the health care context of the country within which they are in. And that's going to have massive amounts of shaping effects on and on and on. And then it also makes that country or that specific context beholden to the
Shubs Upadhyay (41:01)
Mm-hmm.
Jess (41:10)
electronic health record company that is outside of their context. So whereas there are potential opportunities in these places actually to leapfrog, right, maybe we don't need to have huge amounts of investment in AI that's built on the triumphs of an electronic health record.
Shubs Upadhyay (41:23)
Totally.
Jess (41:30)
actually maybe we're going to see huge amount of uptake and benefit from things like ambient AI and things that is running in the background and things that operate on your own device and need none of this in the first place. But in order to get that, that signaling has to be coming from the local context rather than being imposed by, like you say, these big foundations, these big donors who decide that this is what's worked in my place.
Therefore, I'm going to impose it on you, which to me is just smacks of digital colonialism most of the time. And there are more sophisticated ways of thinking.
Shubs Upadhyay (42:08)
And it's definitely why I mean, you know, you touched a lot on the why of this podcast as well. And, you know, I want to have more and more conversations of those examples that have been developed locally and implemented locally. And then suddenly we're, we're like, oh, maybe we should be doing this in the NHS. Like, maybe we should be doing this in, in the US. There's a few examples of some that have been locally developed that US secretaries of state
Jess (42:24)
It's complete.
Shubs Upadhyay (42:31)
visited Eswatini for the EMR for community health workers that they built there and they were like, wow, this is a game changer. Like we should be learning from this. I think it relates to me to another one. one funder on one of the episodes where they said, we're funding you to go in and listen. It's okay that you don't know what the intervention is going to look like. That's fine.
Jess (42:47)
Mm.
Shubs Upadhyay (42:52)
And like, just develop it and co-design it and we'll fund you to do that process. so it might be AI, it might be something else, but you've listened and you've co-designed. And I mean, to me, that seems like a great way to think about this and lives some of the things that you've mentioned here. So one thing I wanted to touch on and you talk about adoption and
Jess (43:09)
Yeah, absolutely.
Shubs Upadhyay (43:15)
and what's what the needs are locally in terms of AI. People might push back and say, well, actually in in these places, there's such a critical shortage of healthcare workers, etc. And it's, you know, there's there's lots of evidence that AI can help bridge this gap.
And therefore like AI is the solution. And so for them, that ticks the box of like, we've seen the problem. And here's the solution coming. So what's your take on that argument?
Jess (43:42)
There are couple of things. mean, my most sort of cynical response is, know, that AI is not a hammer and not every single thing is a nail. sometimes people perceive me as being
very anti AI. I'm not, I'm actually hugely enthusiastic about what its potential is. But my sort of main thought, and it really ties into this argument, is be skeptically optimistic. Okay? So yeah, go in, believe, have as much enthusiasm and optimism for what AI can achieve. And if you think that it is the right
thing that is going to help solve these problems, then by all means go for it. But go in with your eyes wide open about the limitations, about the challenges that you might face and make sure you have those really awkward and difficult conversations upfront. So if we're taking the access one, this comes up even in conversations about in, like you say, in the context of the NHS.
the conversation I often get asked is what's better? Having a nothing or having a low performing algorithm? Are you saying that we should only ever make sure that we deploy algorithms that are like 100 % safe and effective all the time or don't have them at all and therefore let people have nothing? I actually cannot answer that question because I don't know.
Shubs Upadhyay (44:48)
Mm-hmm.
Jess (45:08)
it's going to matter hugely on all of the factors that are involved in that specific context. But all I can say is make sure you've had that conversation. So have it upfront and be aware of the trade-offs that you are making. And be aware of the fact that when you deploy these systems, we see it even in the UK now with automatic triaging, you are creating
a two-tiered system where the people who benefit the most and who probably need the healthcare system the least are the people who have access to a human. And the people who probably need healthcare the most but have least access are going to be the people who get treated by digital solutions and by an algorithm. And morally as a society, because something may be better than nothing, we might be okay with that.
I'm not sure we are, but we might be in certain circumstances. But it's really about making sure we are being honest and recognizing that is what is happening and going in with your eyes open that I think is what matters the most. And then, like I said in my 101, is this really the best solution? And could we take this money that we are going to spend on making an AI
there and do something else with it that might be better off in this particular scenario. And if the answer is no, then by all means, throw AI at it, use it as a giant hammer and see which nails are become smashed flush with the wood.
Shubs Upadhyay (46:47)
Great. You've covered a lot there in your 101. Do you have any, I wanted to break it down because some of these considerations are like from a policy perspective, but maybe could kind of try to get your summary on these. do you have, so I would say like what if, like from a vendor perspective or, know, I'm, I'm a product manager, I'm a designer, I'm a, clinical safety officer, a founder. I'm like, you know, this really resonates, but what can I take away in action?
Like, what can I take to my everyday work and decision making? Do you have a few key nuggets for them?
Jess (47:16)
Yeah, actually it is probably the same for everyone. Biggest sort of summary takeaway is don't fall foul of AI exceptionalism. So anything that you would apply to any other form of technology, apply it to AI and don't believe that it's not necessary. There is no one on earth I know who would be a clinical safety officer who would buy a blood pressure cuff without knowing.
Shubs Upadhyay (47:20)
OK, great.
Jess (47:42)
it was calibrated knowing it was tested, blah, blah, blah. So why would I then do that if I was buying an AI or a digital health solution? And that applies the same then applies also to the policy folk. If I would have a stringent procurement framework or a set of rules about who can buy this and who can sell for other technologies, then I would do the same thing for AI.
Shubs Upadhyay (48:04)
And do you think regulation or procurement is kind of a stronger, I mean, being reductionist here, but if I use a model of thinking of like regulation being, and it's not always a stick, but regulation kind of is a forcing function sometimes to make you think about these things in right way, ultimately to deliver hopefully a great product that's well evidenced, right? And do some of that. And that's how, that's how the blood pressure cough gets over the line, right? And regulation made all of those things happen, right?
And in some ways, it's a stick. there's the regulation, like how do you see regulation as an enabler of making sure ethical principles and all of these things get upheld? And then kind of there's the procurement side of like, how do you elevate and make survive? And we talked about, you know, evolutionary pressures earlier, you know, ultimately for the health outcomes that we want, how do you make survive the vendors who've actually, and how do they win the contracts?
rather than the ones who cut corners and like are able to say, hey, we're like, you know, we're super cheap, but like they cut corners. So like, how do you, how do you elevate the ones who've lent in to quality, to evidence, to equitable approaches to, to their ethical, living their ethical principles.
Jess (49:15)
So first on the regulation thing, the point really is, you said it in your question, frame regulation as an enabler. Regulation at the moment has a really bad rep. It's all what you get in the rhetoric and it is in the UK, but it's everywhere, pro innovation regulation. We saw this again, it was one of those things that came up in the AI action plan.
was it says in there something along the lines of we have an advantage because we want to be more regulatory flexible than other places. This doesn't compute. Regulation. There is, has a bad rep. know, this is like Taylor Swift in 2017. this is not, there is no evil thing inherent in the reputation of regulation, right?
Shubs Upadhyay (49:59)
Hehehehe
regulation.
Jess (50:04)
we can think of regulation as being an inherent good. What we can design is regulatory friendly innovation rather than innovation friendly regulation and make sure that the regulation is designed in a way that it is an enabler, not a stifler. But that is all in the design of the regulation itself, not in the concept of regulation existing. So that is sort of point number one. The other ones are
It's just boring. We like to talk about AI ethics and governance and all of these things as if it is entirely novel concept. It's not we know how to do this thing. It's contracting procurement and licensing and making sure that we are using all of those levers to get the outcomes that we want. You don't want to use
You don't want this product to be used for people it hasn't been tested on. That's a licensing thing. Okay, make sure it's in the end user license agreement. You can't use it off label. You can't use it off the body of people that it was tested on. You want to make sure that the outcomes are what they determined. That's in the contract. Okay, if your performance starts to drift,
and you don't do what you said that you were going to deliver, then I have the right to terminate your contract. With procurement, that is all about how you design the procurement framework. Okay, we want to make sure that we elevate these people and we elevate these. Then we start putting in those procurement frameworks. These are the things I want you to demonstrate. And you make sure that people are scored appropriately. And then you do things on top of all of that.
like making sure contracts are put in the public domain, making sure that they are auditable, making sure that people can question them, that they can be FOI'd, all of that type of stuff. It's all phenomenally boring. But there is a great, great paper that talks about infrastructure that basically says we shouldn't dismiss it because it is boring and actually some of the greatest, most successful and beautiful things.
come in The Boring. So let's have more conversations about The Boring.
Shubs Upadhyay (52:04)
That sounds great. And so all of these things that you've mentioned seem like, you know, if they're put into place, could be great, right? If we come full circle to what you said at beginning, which was we've gone backwards, and now everything's to do with economic growth. Do think that's going to happen? Like the things you've set out? Because procurement won't like that's not like, according to what you said at the beginning.
Jess (52:20)
Hmm.
Shubs Upadhyay (52:29)
It's now so three to five years ago, like that was the I guess like a great opportunity to like it fit with the rhetoric, it fit what everyone was talking about. Everyone was like was ethics washing the hell out of themselves. And so that seemed like the place to do it, right. And now it's all about economic growth. So I worry that these things won't come into procurement.
Jess (52:47)
Yeah, I I worry about that too. And do I think it will happen?
Yes and no, but I honestly think framing all of this stuff as being things that fall into things like procurement, contracting and licensing, as opposed to ethics, might help it happen. It might help it because it makes it seem more in line with the current sort of state of play and fix.
Shubs Upadhyay (53:15)
And this is
your skeptically optimistic take, right? Awesome. Perfect. So this sounds great. Jess, I want to wrap up, but before you talked about the Yale Digital Ethics Lab, what are you guys doing? Tell us about that. That would be great to hear about.
Jess (53:18)
Yes, exactly, this is my skeptic.
Yeah.
So we are the Digital Ethics Centre. We look at GELSI depending on whether you say GIF or GIF. But it is the governance, ethical and legal social implications of technologies at large. And we look at basically how do you design, the key word is design, those better digital future, essentially.
Shubs Upadhyay (53:54)
And so
not just within health, but actually across, yeah.
Jess (53:56)
No, so I was about
to say there are different pillars of research, they all sort of fall within the future of. So I run the future of health pillar, but we also have the future of content, we have the future of reality, we have the future of regulation, but there are a bunch of different things that we look at. I just happen to be the health nerd.
Shubs Upadhyay (54:18)
And a couple of months ago, you had this session in Venice, right? You brought a bunch of experts from globally together to talk specifically about healthcare and AI and healthcare. How did that go?
Jess (54:25)
Mm-hmm.
It was great. Yes. So we hosted an event called the something along the lines of global health in the age of AI. And what we tried to do was break down silos in much the same way that we have a little bit in this conversation. We've just not done it so explicitly that I think very often in conversations about ethics of technology or indeed about technology in general, you get the techies.
over here, then you get the ethicists over there, then you maybe get the lawyers in a different room, and everybody sort of sits and has their own conversations and they're all talking to their friends. We tried to make people talk to their neighbors as well as just their friends. And then we also tried to make sure that we had people from as wide a geographical representation as we possibly could to try and sort of break down that siloed thinking. And it was great. Everybody had a nice time. We were on a private island off the coast of Venice, so I'm not sure.
that could have been bad, but we had a lot of very productive conversations and you should see the consensus paper coming out in the next couple of months.
Shubs Upadhyay (55:30)
We'll definitely keep an eye on that. And when it comes out, we can add it to the show notes as well. How can people find you to see the work that you're doing and potentially reach out to collaborate with you, if that's what you're looking for, by the way. I assume that you are.
Jess (55:41)
Yes,
always, always looking for collaborations. I exist in a lot of places on the internet. I no longer exist on this website, formerly known as Twitter, but I am on Blue Sky. I am on LinkedIn and my email is public. So it's just jessica.morley at yale.edu. You can contact me through any of those different channels.
Shubs Upadhyay (56:02)
And you kind of have, you post articles regularly. So you've got this kind of, you've got your health data nerd stuff that you post as well, which is very informative. So yeah, I've learned a lot from you over the years and the things that you rewrite about and speak about. So I really look forward to the stuff that you're going to be doing at Yale. I wanted to quickly mention my quick takeaways that I've taken. So we've generally gone backwards in terms of like the state of the industry.
Jess (56:24)
Mm-hmm.
Shubs Upadhyay (56:28)
where three to five years ago we were really pro-ethical and this was seen as a strategic advantage, both from a government perspective, like layer perspective, but also from a vendor perspective. You see a shift now because of the macroeconomic climate. Things like focus very much and like the AI opportunity being a case, like a case, a good case study is very much focused on like, we need this to support economic growth.
Second one, build ethics into your everyday kind of product thinking for vendors. Build it into your everyday decision making of like how we're building a good product and can delight our customers and users. Three, think critically about, especially if you're a decision maker of like, know, why is, you know, what's the, I think the one I took away the most was like, what are the bigger health outcomes we want to achieve?
is AI really the right thing for this? who decided this? also, there was something around what resources might be used instead? What's the opportunity cost of this? I think that was an important one. And I think the fourth one, which is a good takeaway generally for mindset is
be sceptically optimistic. Is that a good summary of the key takeaways? I mean, those are what I've taken away the most from talking to you today, Jess.
Jess (57:39)
Yes.
Definitely. Thanks so much for the conversation.
Shubs Upadhyay (57:49)
Jess, it's been really, really insightful. I look forward to seeing work that you're doing. I'm looking forward to following more of your hot takes. Thank you, for your insights and helping us to kind of have more critical thinking into our everyday work. Thank you so much.
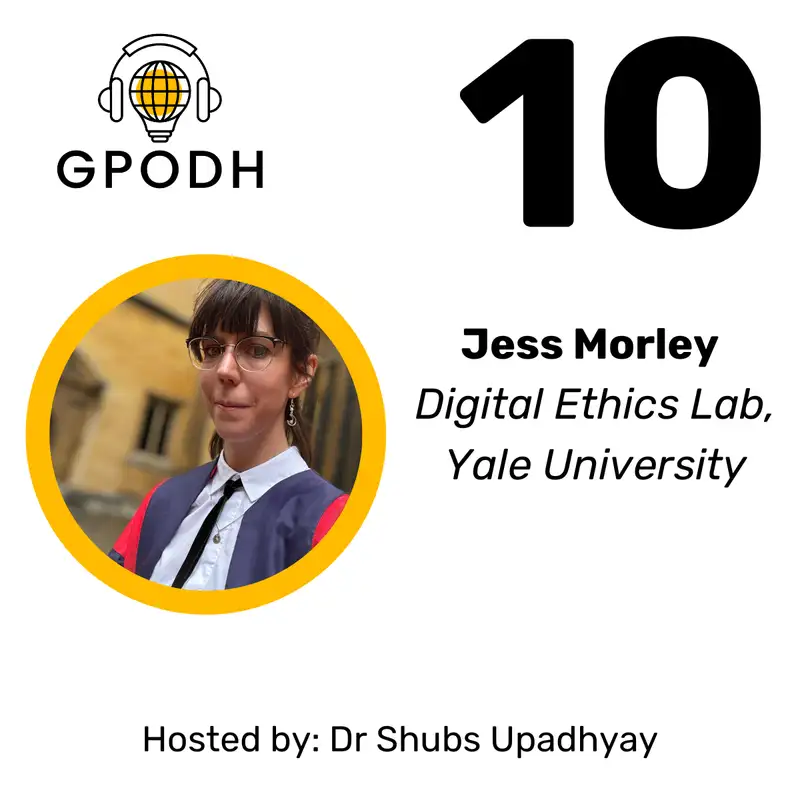